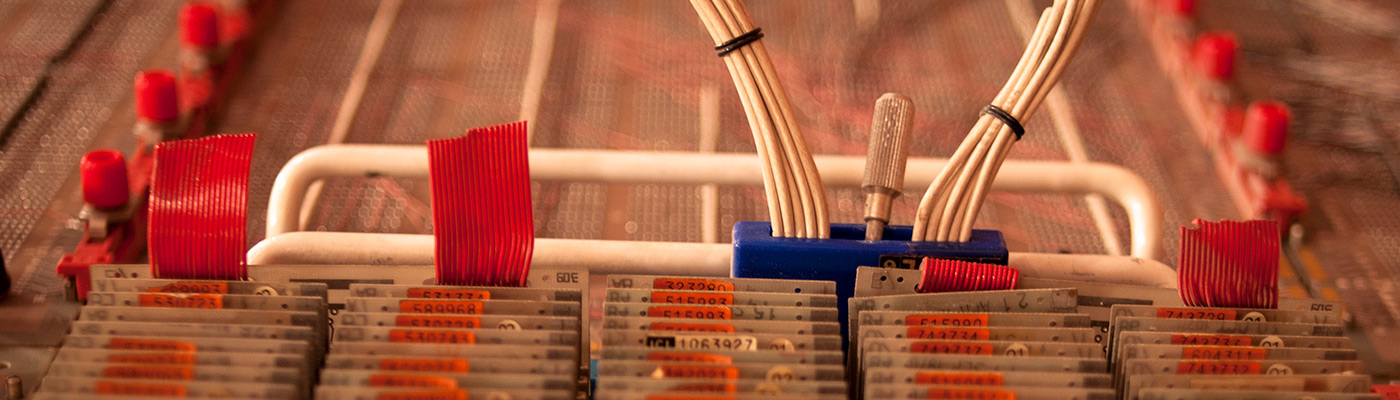
Research themes
Our research tackles some of society's greatest challenges; from making sense of the data deluge to the security of communications and figuring out how to live alongside intelligent robotic systems.
We see research in computer science as a virtuous circle, where we investigate the fundamental nature of new computing paradigms and feed it back into our work to create real-world impact.
Our research is structured to contribute meaningfully across several broad themes:
Artificial intelligence
We are forging a path toward seamless integration of intelligent systems into our natural environment.
Data science
Our expertise spans the full data science lifecycle: from information management to bio-health informatics.