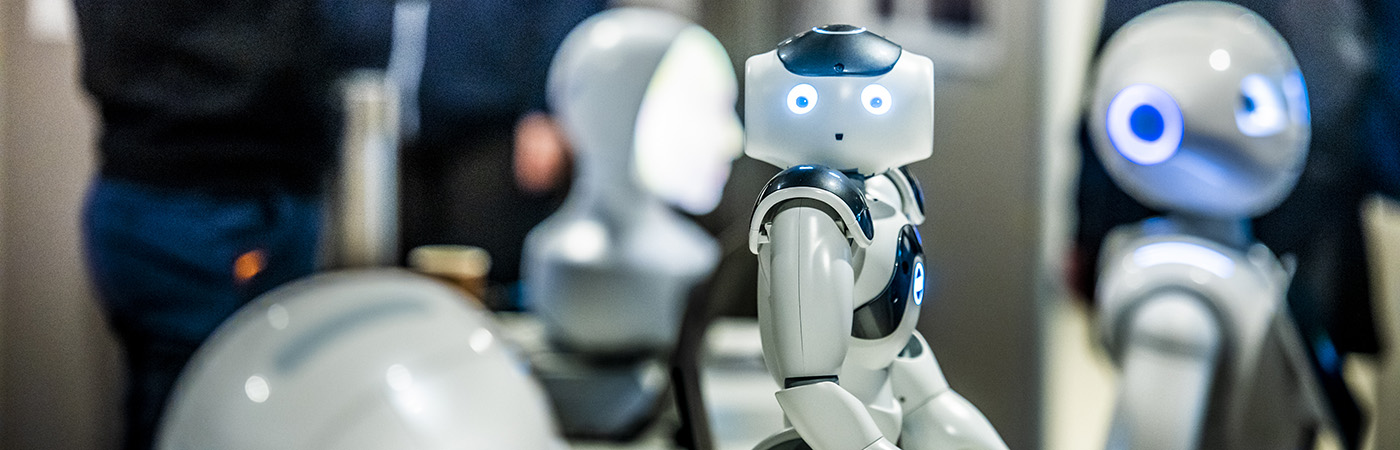
Undergraduate courses
We have an array of computer science course options available, each of which are designed to provide a thorough grounding in the subject.
Whether your interests lie in human-computer interaction, AI or software engineering, there is an undergraduate course option to suit you.
You can choose a three-year BSc course to get to grips with your chosen subject, or a longer four year BSc programme - including a year with industrial experience - to broaden your experience further.
Explore our available courses below.